The Transformation of SaaS Through White Label AI
The software-as-a-service (SaaS) industry is witnessing a profound shift with the integration of artificial intelligence capabilities. AI white label SaaS represents a significant breakthrough, allowing businesses to leverage sophisticated AI functionalities without building them from scratch. This approach enables companies to offer AI-powered solutions under their own brand, creating unique value propositions while minimizing development costs. The white label AI market has grown exponentially as organizations seek to enhance their service offerings with intelligent automation, conversational interfaces, and predictive analytics. According to recent industry reports from Gartner, AI software market is projected to reach $135 billion by 2025, with white label solutions accounting for a substantial portion of this growth. Companies utilizing white label AI solutions can focus on their core business while still providing cutting-edge technological experiences that meet customer demands for intelligence-driven interactions, as illustrated by successful implementations in AI call center operations.
Understanding the Core Components of AI White Label SaaS
At its foundation, AI white label SaaS combines several technological components that work together to create powerful, rebrandable solutions. These typically include natural language processing (NLP) engines, machine learning algorithms, data analytics frameworks, and customizable user interfaces. The backbone of these systems often incorporates large language models (LLMs) that enable human-like conversations and text generation capabilities. Voice synthesis technology, like those discussed in Callin.io’s guide to voice synthesis, transforms text into natural-sounding speech for applications like AI phone agents. Integration capabilities allow these systems to connect with existing business tools such as CRMs, calendaring systems, and communication platforms. White label providers typically offer customization options for branding, workflow design, and AI behavior configuration, allowing resellers to tailor the solution to their specific market needs without requiring deep technical expertise in AI development.
Revenue Models and Business Opportunities
The financial landscape for AI white label SaaS presents diverse monetization pathways for both providers and resellers. Typical revenue structures include subscription-based models with tiered pricing based on feature access and usage volume. Many providers offer revenue-sharing arrangements where resellers receive a percentage of recurring revenue from their clients. Some white label solutions implement transaction-based pricing, particularly for AI-powered communication tools like those described in Callin.io’s AI calling business guide. The market opportunity for resellers is substantial, with relatively low entry barriers compared to developing proprietary AI solutions. For entrepreneurs interested in this space, starting an AI calling agency can be particularly lucrative, as voice AI applications continue to see strong demand across industries. White label AI also enables current SaaS businesses to expand their service portfolios horizontally, adding new revenue streams without significant R&D investments. This creates a win-win situation where providers scale through their partner network while resellers leverage cutting-edge technology without the associated development costs.
Voice AI: The Frontier of White Label AI Solutions
Among the most rapidly expanding segments in the AI white label SaaS market is voice AI technology, which enables natural-sounding, conversational interactions between computers and humans. These solutions have transformed customer service operations by providing AI voice assistants for FAQ handling and comprehensive support systems. The technology has evolved beyond simple command recognition to facilitate complex, contextual conversations that can understand intent, remember previous interactions, and provide personalized responses. White label voice AI platforms like those offered through Callin.io’s AI voice agent solutions allow businesses to deploy sophisticated phone agents that can handle appointment scheduling, sales calls, customer inquiries, and more. The integration capabilities with telephony systems through providers like Twilio (or Twilio alternatives) enable seamless deployment across existing communication channels. For businesses seeking to implement voice AI, options range from complete white label solutions to customizable frameworks that can be tailored to specific industry requirements, creating unique competitive advantages through specialized AI communication capabilities.
Competitive Analysis: Leading White Label AI Providers
The AI white label SaaS marketplace features several distinguished providers, each with unique strengths and specializations. SynthFlow AI, featured in Callin.io’s SynthFlow AI white label review, excels in conversational AI for business communications with natural-sounding voice agents. Air.ai, another contender highlighted in Callin.io’s Air AI analysis, focuses on multilingual capabilities and seamless integration with existing business systems. Vapi.ai, discussed in Callin.io’s Vapi AI white label guide, stands out for its developer-friendly API structure and flexible deployment options. Bland AI, explored in Callin.io’s Bland AI white label review, offers robust solutions for automated customer engagement across industries. Retell AI, with alternatives examined in Callin.io’s Retell AI alternatives, provides distinctive voice cloning capabilities. When selecting a white label AI partner, businesses should evaluate factors including customization depth, integration capabilities, pricing structures, voice quality, and ongoing support services. The most successful providers typically offer comprehensive onboarding, training resources, and continuous improvement of their AI models based on real-world performance data.
Industry Applications and Use Cases
The versatility of AI white label SaaS enables implementation across diverse sectors, with particularly strong adoption in specific industries. In healthcare, AI phone agents are revolutionizing patient engagement through AI calling bots for health clinics that manage appointments, follow-ups, and routine inquiries. The real estate sector has embraced AI calling agents for property management that handle tenant communications, property showings, and lead qualification. Retail businesses implement AI-powered solutions to reduce cart abandonment rates through timely follow-ups and personalized recommendations. Financial services firms deploy white label AI for client onboarding, routine transaction support, and preliminary financial guidance. The hospitality industry utilizes these technologies for reservation management and guest services enhancement. Small businesses across sectors benefit from AI voice receptionists that provide professional call handling without dedicated staff. Educational institutions employ AI communication tools for enrollment processes and student support services. Each implementation showcases how white label AI solutions can be customized to address industry-specific challenges while maintaining brand consistency and service quality.
Technical Implementation and Integration Challenges
Deploying AI white label SaaS solutions requires careful technical consideration to ensure seamless operation with existing business systems. Integration with telecommunication infrastructure often presents the first hurdle, requiring partnerships with SIP trunking providers to enable voice communications. Businesses must address data security and compliance requirements, particularly in regulated industries where customer information protection is paramount. API compatibility between the white label AI platform and existing CRM, ERP, or customer service systems demands thorough evaluation during the selection process. Voice quality optimization presents another challenge, with considerations for factors like text-to-speech technologies that impact perceived professionalism. Customization capabilities vary significantly between platforms, with some requiring prompt engineering expertise to achieve desired outcomes. Scalability concerns must be addressed, especially for businesses anticipating rapid growth in AI interaction volume. Implementation timelines typically range from several weeks to months, depending on complexity and customization requirements. Successful deployments generally involve phased approaches, beginning with limited use cases before expanding functionality based on performance data and user feedback, as outlined in Callin.io’s guide to creating AI call centers.
Custom Branding and Personalization Options
The essence of AI white label SaaS lies in its ability to seamlessly blend with a company’s existing brand identity while delivering sophisticated AI capabilities. Effective white label solutions offer comprehensive branding options including customizable voice personalities that align with brand values—professional, friendly, authoritative, or casual. Visual interface elements like dashboards, reports, and customer-facing portals can be styled with company colors, logos, and design elements to maintain brand consistency. Communication templates for emails, SMS, and voice interactions can be tailored to reflect the company’s tone and messaging standards. Some providers offer custom AI voice development, allowing businesses to create distinctive vocal identities for their AI agents. Domain customization enables the AI solution to operate under the reseller’s web domain, maintaining brand integrity throughout the customer journey. For businesses requiring specialized industry vocabulary or knowledge bases, white label solutions typically support custom data integration to enhance AI performance in specific contexts. The most effective implementations achieve a level of personalization where end users may not distinguish between the AI service and the company’s human-delivered services, creating a cohesive brand experience across all customer touchpoints.
Measuring Success: Analytics and Performance Metrics
Effective implementation of AI white label SaaS requires robust performance tracking to justify investment and guide optimization efforts. Key performance indicators typically include conversation completion rates, which measure how effectively the AI handles interactions without human intervention. Customer satisfaction metrics captured through post-interaction surveys provide insight into quality perception. Resolution time comparisons between AI and human agents help quantify efficiency gains. Cost savings calculations consider reduced staffing requirements against the SaaS subscription fees. Revenue generation metrics track direct sales or appointments set by AI agents, particularly relevant for AI appointment setters and sales applications. Comprehensive analytics dashboards, such as those available through Callin.io’s task dashboard, enable businesses to monitor these metrics in real-time. Conversation analysis tools identify common customer inquiries, points of confusion, or service gaps that require attention. Advanced platforms incorporate machine learning to continuously improve performance based on interaction data. For businesses serving multiple market segments, comparative analysis across different customer groups helps refine AI behavior for maximum effectiveness with each audience. Regular performance reviews should inform ongoing customization and prompt refinement to address any identified weaknesses in the AI’s conversational capabilities.
Customer Experience and Perception Management
Implementing AI white label SaaS solutions requires careful attention to customer perception and experience quality. Transparency about AI usage remains a contentious issue, with approaches ranging from explicit disclosure to seamless integration that mimics human interaction. The quality of conversational design significantly impacts customer satisfaction, with natural dialogue flows and contextual understanding creating more positive experiences. Error handling capabilities, particularly the AI’s ability to recognize its limitations and escalate to human agents when necessary, proves critical for maintaining trust. Many successful implementations use AI phone assistants as complementary to human teams rather than complete replacements. Customer feedback mechanisms should be integrated into the AI system, allowing continuous improvement based on real user experiences. Voice tone and personality consistency contribute significantly to brand perception, making voice agent selection an important consideration. Cultural sensitivity in AI responses requires attention, particularly for businesses serving diverse customer populations. Companies achieving the highest customer satisfaction typically invest in ongoing conversation design improvements and regular performance evaluations, treating the AI system as an evolving asset rather than a static implementation. When properly executed, white label AI can enhance rather than diminish the perceived personalization of customer interactions.
Scaling AI Operations: From Pilot to Enterprise Implementation
The journey from initial AI white label SaaS experimentation to full-scale deployment follows a structured progression that minimizes risk while maximizing adoption success. Starting with proof-of-concept implementations focusing on limited, well-defined use cases allows organizations to validate performance before broader investment. Proper infrastructure planning, including evaluation of telephony requirements and integration points, establishes a solid foundation for growth. Staff training programs ensure that employees understand how to cooperate with AI systems, particularly for handling escalations from AI phone services. Data feedback loops must be established to capture interaction information that drives continuous improvement. Governance frameworks for AI behavior management, including approval processes for conversational updates and performance monitoring protocols, become increasingly important as deployment scales. Organizations successfully navigating this growth typically establish phased rollout plans with clear success metrics at each stage. Resource allocation between AI and human components requires regular reassessment based on volume fluctuations and complexity patterns. Leading enterprises create cross-functional teams responsible for AI governance, including technical, customer experience, compliance, and business strategy stakeholders. Documentation of best practices and lessons learned throughout the scaling process creates valuable organizational knowledge that supports future AI initiatives across the business.
Ethical Considerations and Responsible AI Deployment
The implementation of AI white label SaaS requires thoughtful consideration of ethical implications and responsible usage guidelines. Transparency with customers about AI interactions represents a fundamental ethical principle, with clear disclosure about when and how AI is being used in communications. Data privacy protections must exceed minimum legal requirements, with special attention to personally identifiable information and sensitive customer data. Bias mitigation in AI responses demands ongoing attention, particularly for conversational systems that may inadvertently perpetuate stereotypes or discrimination. Human oversight mechanisms should be established to monitor AI interactions and intervene when necessary. Service accessibility considerations ensure that AI systems don’t disadvantage specific customer groups through design choices. Respect for user autonomy includes providing options to connect with human representatives when preferred. For AI cold calling applications, particular care must be taken to respect contact preferences and avoid creating negative experiences. Organizations should establish and document AI ethics policies that guide implementation decisions and provide governance frameworks. Regular ethical impact assessments help identify potential issues before they affect customers. Leading organizations in this space recognize that ethical AI deployment represents both a moral imperative and a business advantage, as customer trust increasingly depends on responsible technology usage.
Competitive Differentiation Through AI Customization
In the crowded AI white label SaaS marketplace, creating distinctive value propositions through customization provides critical competitive advantages. Industry-specific knowledge integration enables white label resellers to tailor AI capabilities to unique vertical market needs, such as specialized vocabulary, regulatory requirements, or common customer scenarios. Personalization capabilities that adapt AI responses based on customer history and preferences create more engaging interactions than generic approaches. Integration with proprietary systems and workflows allows organizations to embed AI capabilities within their unique operational frameworks. Custom response handling for brand-specific situations enables AI systems to perfectly reflect company policies and service approaches. Voice personality development, as highlighted in Callin.io’s AI voice assistant guide, establishes distinctive brand experiences through tone, speaking style, and conversational nuances. Advanced resellers develop proprietary dialogue frameworks that structure conversations in ways that reflect their unique service methodology. Specialized analytics dashboards provide insights specifically relevant to the business model and customer base. Organizations achieving the strongest market differentiation typically invest in creating unique combinations of these customization elements rather than focusing on a single aspect, resulting in AI implementations that competitors cannot easily replicate through standard white label offerings alone.
Future Trends in AI White Label SaaS
The AI white label SaaS sector continues to advance rapidly, with several emerging trends poised to reshape the industry. Multimodal AI capabilities that combine voice, text, and visual interaction channels will create more comprehensive customer experiences. Enhanced personalization through deeper behavioral analysis will enable AI systems to adapt more effectively to individual communication styles and preferences. Industry-specific AI models pre-trained on vertical market data will improve performance in specialized domains from the outset. Integration with emerging LLM technologies will enhance conversational capabilities and contextual understanding. Emotion recognition and sentiment analysis will enable more empathetic AI responses based on detected customer states. Advanced accent and dialect handling will improve accessibility across diverse user populations. Voice cloning technologies like those explored by platforms featured in Callin.io’s AI voice comparisons will become more sophisticated and ethically implemented. Self-learning capabilities will reduce the need for manual optimization over time. Specialized AI applications for complex processes beyond basic customer service, such as AI sales representatives handling complex negotiations, will expand the market. Regulatory frameworks specifically addressing AI communications will mature, creating clearer compliance guidelines. Organizations positioning themselves at the forefront of these trends will find competitive advantages as the white label AI market continues to evolve and customer expectations for intelligent interactions increase.
Integration With Business Communication Ecosystems
Successful AI white label SaaS implementation requires thoughtful integration with existing business communication frameworks. Omnichannel connectivity, as explored in Callin.io’s omnichannel guide, ensures consistent AI experiences across voice, text, email, and messaging platforms. CRM integration enables AI systems to access customer histories, preferences, and previous interactions for more personalized engagement. Calendar systems connectivity, particularly important for AI appointment scheduler applications, allows automated booking and rescheduling capabilities. Workflow automation platform integration enables AI interactions to trigger appropriate business processes based on conversation outcomes. Knowledge base connections provide AI systems with access to current product information, policies, and procedural guidelines. Telephony infrastructure integration through SIP trunking or specialized providers enables high-quality voice communications. Support ticketing system connectivity ensures smooth handoffs between AI and human agents when escalation is required. Analytics platform integration provides comprehensive performance visibility within existing business intelligence frameworks. Organizations achieving the most successful ecosystems take an architectural approach to integration, mapping data flows and interaction points comprehensively rather than creating point-to-point connections. This holistic integration strategy ensures that AI becomes a seamless component of the business communication landscape rather than an isolated technology implementation.
Cost-Benefit Analysis and ROI Calculations
Investing in AI white label SaaS requires rigorous financial analysis to justify expenditure and measure returns. Direct cost savings typically include reduced staffing requirements for routine communications, lower training expenses, and decreased turnover-related costs. Operational efficiency improvements manifest as increased handling capacity, extended service availability beyond business hours, and faster response times. Revenue enhancement opportunities emerge through improved lead response rates, increased appointment setting efficiency through AI scheduling systems, and enhanced customer retention due to consistent service quality. Implementation costs encompass platform subscription fees, integration expenses, customization requirements, and training for staff who interact with the AI system. Ongoing maintenance considerations include prompt refinement, performance monitoring, and periodic system updates. ROI timelines vary significantly by implementation scope and industry, typically ranging from 6-18 months for well-executed projects. Phased implementation approaches often yield faster returns for specific use cases while building toward comprehensive deployment. Organizations should establish clear baseline metrics before implementation to accurately measure impact. The most sophisticated ROI analyses include qualitative factors such as customer experience improvements and brand perception enhancements alongside quantitative financial calculations. This comprehensive approach provides a more accurate picture of the total value created through AI white label adoption.
Building a Reseller Strategy for White Label AI
For businesses considering entering the AI white label SaaS market as resellers, strategic positioning and operational preparation prove essential for success. Market segmentation analysis should identify specific industries or business types where AI communications offer particular value, such as appointment-dependent services or high-volume customer support operations. Value proposition development must articulate clear benefits beyond generic AI capabilities, focusing on specific problems solved for the target market. Service packaging and pricing strategies typically combine platform access with value-added services like customization, training, and ongoing optimization. Sales and marketing approaches should educate potential clients about AI capabilities while addressing common concerns regarding implementation complexity and customer acceptance. Technical partnership management with the white label provider ensures access to product roadmaps, early feature releases, and priority support. Resources for AI resellers highlight the importance of developing internal expertise to provide knowledgeable pre-sales consultation and implementation support. Operational infrastructure requirements include support systems, billing mechanisms, and account management processes. Successful resellers typically create showcase implementations that demonstrate their specific expertise and customization capabilities. Performance monitoring frameworks should track both financial metrics and customer success indicators to identify opportunities for service improvement. The most effective reseller strategies combine technology access with specialized knowledge and support services, creating value that extends beyond merely reselling platform access.
Case Studies: Successful AI White Label Implementations
Examining real-world AI white label SaaS deployments provides valuable insights into success factors and implementation approaches. A mid-sized medical practice implemented an AI voice assistant for medical office management, reducing appointment scheduling staff requirements by 60% while extending booking availability to 24/7 operation. A regional real estate agency deployed white label AI phone agents for property inquiries, increasing lead response rates by 85% and property viewings by 37% through immediate inquiry handling. A retail chain implemented conversational AI for customer service, reducing call abandonment rates from 23% to under 5% while maintaining high customer satisfaction scores. A financial services firm utilized white label AI cold calling technology for appointment setting, achieving a 42% increase in consultation bookings while reducing acquisition costs by 28%. A multinational company deployed white label AI phone consultants across multiple regional offices, creating consistent customer experiences while supporting local language requirements through multilingual AI capabilities. Common success factors across these implementations include clear use case definition, thoughtful customization to specific business requirements, comprehensive testing before full deployment, and continuous optimization based on performance data. Organizations sharing these success stories frequently emphasize the importance of setting appropriate customer expectations and providing fallback options to human assistance when needed.
Best Practices for AI Voice Agent Deployment
Implementing AI white label SaaS voice agents requires adherence to established best practices to maximize success rates and customer satisfaction. Conversation design should prioritize natural dialogue flows with appropriate turn-taking, conversational markers, and logical progression. Voice selection must align with brand identity and customer expectations, considering factors like perceived gender, accent, and tonal qualities as discussed in Callin.io’s AI receptionist guide. Prompt engineering, covered in Callin.io’s prompt engineering guide, requires careful crafting of AI instructions to ensure desired behaviors and responses. Testing protocols should include diverse customer scenarios, edge cases, and potential misunderstandings to identify weaknesses before public deployment. Escalation pathways need clear definition, ensuring smooth transfers to human agents when AI capabilities are exceeded. Customer education about interacting with AI systems often improves satisfaction and completion rates. Performance monitoring should combine automated metrics with conversation reviews by human quality assurance personnel. Continuous improvement processes must incorporate both technical refinements and conversational enhancements based on real-world interactions. System redundancy planning ensures service continuity during technical disruptions. Documentation of AI behaviors, capabilities, and limitations provides important reference material for both implementation teams and users. Organizations following these best practices typically achieve higher success rates in initial deployment and more sustainable long-term performance from their AI voice agent implementations.
The Future of Call Centers: AI-Powered Communication Hubs
The transformation of traditional call centers into AI white label SaaS-powered communication hubs represents one of the most significant applications of this technology. Hybrid staffing models combining AI agents and human representatives create optimal balances between efficiency and personalized service. Intelligent routing systems direct inquiries to either AI or human agents based on complexity, customer preference, and historical interaction data. AI for call centers enables 24/7 service availability without premium labor costs for overnight staffing. Quality monitoring becomes more comprehensive through automated analysis of 100% of customer interactions rather than small sample reviews. Agent augmentation tools provide real-time guidance and information access for human representatives handling complex cases. Predictive analytics identify potential customer issues before they escalate, enabling proactive outreach. Seamless channel transitions allow conversations to move between voice, text, and digital platforms while maintaining context. Workflow automation triggered by conversation outcomes reduces manual processing requirements. Industry-specific implementations like Vicidial AI agent integration combine existing call center infrastructure with new AI capabilities. Organizations achieving the greatest success in this transformation focus on creating complementary relationships between AI and human capabilities rather than viewing implementation as a simple replacement strategy. The resulting communication hubs deliver higher customer satisfaction, operational efficiency, and data-driven insights than traditional call center models, creating sustainable competitive advantages.
Try Callin.io: Your Partner for AI-Enabled Business Communications
If you’re ready to harness the power of AI white label SaaS for your business communications, Callin.io provides the ideal platform to get started. Our comprehensive solution allows you to deploy sophisticated AI phone agents that handle incoming and outgoing calls with natural conversations, automating everything from appointment scheduling to answering frequently asked questions and even closing sales opportunities. The technology seamlessly integrates with your existing business systems while maintaining your brand identity.
Getting started with Callin.io is straightforward and risk-free. Create your free account today to access our intuitive interface for configuring your AI agent, with complimentary test calls included to experience the technology firsthand. Our task dashboard provides complete visibility into all AI interactions, helping you monitor performance and identify optimization opportunities. For businesses requiring advanced functionality like Google Calendar integration or built-in CRM capabilities, our subscription plans start at just $30USD monthly, offering exceptional value compared to traditional staffing costs.
Don’t let your business communications remain stuck in the past while competitors advance with AI technology. Discover Callin.io today and transform how your business handles phone conversations with cutting-edge AI that works for you around the clock.
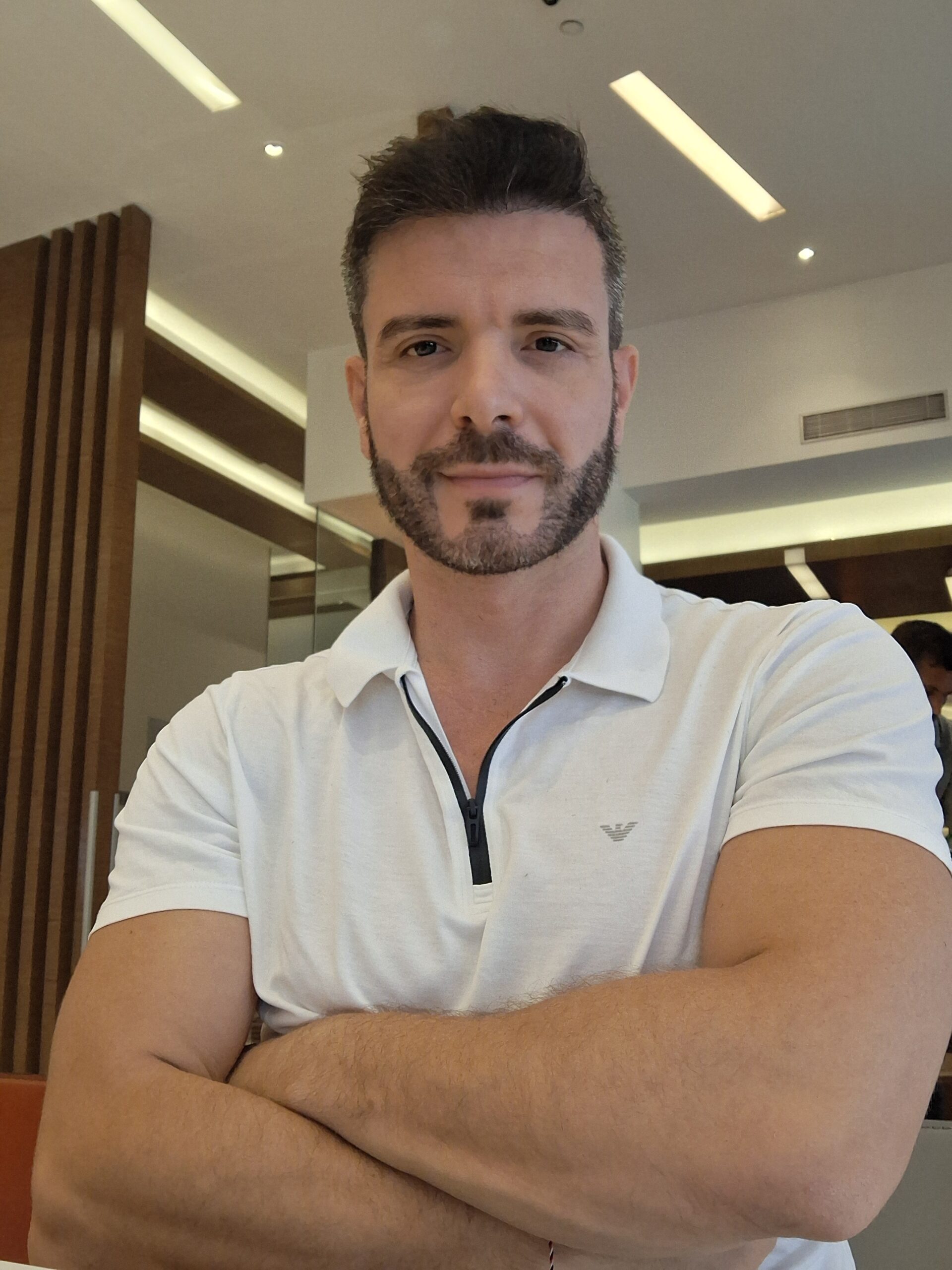
Helping businesses grow faster with AI. 🚀 At Callin.io, we make it easy for companies close more deals, engage customers more effectively, and scale their growth with smart AI voice assistants. Ready to transform your business with AI? 📅 Let’s talk!
Vincenzo Piccolo
Chief Executive Officer and Co Founder